A common mistake made by researchers, project managers, and other stakeholders looking at data quality in a multi-sourced world is concluding that Supplier A is bad quality because most of the interviews rejected were from Supplier A.
What follows may strike you as basic math.
Take an example survey where you’ve collected 1,000 completes from four different providers, and after review, you toss out 10% of those interviews for quality issues. Here’s the distribution:
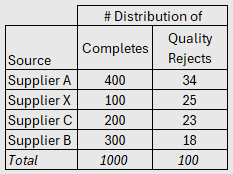
If you say Supplier A is the “worst” supplier, or the one driving your data quality issues, you are incorrect. Supplier A might have the most interviews tossed out, but it is under-indexed for rejections. Supplier X is your real problem, with a rejection rate 2.5x the overall rate.
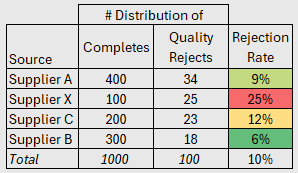
One More Thing
While you’re here though, I’ve got a hotter take on this topic. Let’s take another example survey, where you have collected 1,000 interviews from 6 different sources, and upon review you decide to toss out 250 of the responses. That’s 25%! This is a lot of bad data, there must be a supplier or two driving these rates. Here is the distribution:
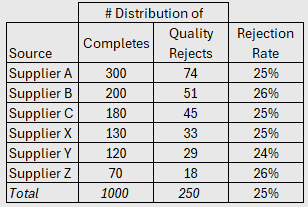
The distribution of completed interviews matches the distribution of rejections. More times than not, this indicates that the survey design is the problem. Take a look at the questionnaire, is there a large grid with 5+ choices where you rejected people for straightlining? Were there four open-end questions late in a twenty minute survey, and those responses were lacking or even nonsensical? When there is good distribution of responses across diverse, vetted sources and you have a high toss-out rate that is proportionate to the completes, it’s unlikely all these sources provided high levels of bad data. You are better served examining the survey experience and if that generated “bad” responses in the field.
The data quality conversation often involves researchers pointing fingers at sample providers for bad respondents, and sample providers crying “survey design” in response. Given that most projects are multi-sourced today, transparently or not, this simple analysis can help partners address what drove sub optimal results, and more quickly resolve it for the next project.